The Honda Prize 2019 Awarded to Dr. Geoffrey Hinton, Professor Emeritus, the University of Toronto and Chief Scientific Adviser, Vector Institute
-Pioneering research that paved the way to the application of artificial intelligence (AI) in a broad range of areas and contributed to its practical application-
September 20, 2019, Japan
Honda Foundation, the public interest incorporated foundation established by Soichiro Honda and his younger brother Benjiro and currently led by President Hiroto Ishida, is pleased to announce that the Honda Prize 2019 will be awarded to Dr. Geoffrey Hinton, Professor Emeritus of the University of Toronto and Chief Scientific Adviser of the Vector Institute for his pioneering research in the field of deep learning*1 in artificial intelligence (AI) and his contribution to practical application of the technology.
The Honda Prize, established in 1980 and awarded once each year, is an international award that recognizes the work of individuals or groups generating new knowledge to drive the next generation, from the standpoint of eco-technology*2. Dr. Hinton has created a number of technologies that have enabled the broader application of AI, including the backpropagation algorithm*3 that forms the basis of the deep learning approach to AI. AI is expected to play an important role not only in the advancement of science and technology but also in resolving many different global issues that humankind must address in the areas of energy and climate change. The Prize will be awarded to Dr. Hinton for his outstanding achievements worthy of the highest recognition.
This year marks the 40th award of the prize. The presentation ceremony will be held on November 18, 2019 at the Imperial Hotel Tokyo, at which Dr. Hinton will be presented the medal, the certificate and an honorarium of 10 million yen.
<Research by Dr. Geoffrey Hinton>
When AI was in its infancy in the 1960s, the dominant paradigm involved using symbolic, hand-coded representations of knowledge that could be processed by a computer using rules of inference. The biologically inspired alternative to symbolic AI was artificial neural networks that learned to use the activity patterns of large sets of neurons as distributed representations of data. The neural network paradigm was largely unsuccessful until 1986 when Dr. Hinton and his collaborators introduced the backpropagation algorithm and demonstrated that neural networks could learn distributed representations of concepts from symbolic data. Currently, this technology is the standard method for learning neural networks, with the number of references in academic papers to date exceeding 60,000. However, the practical applications were limited because the datasets were too small and computers were too slow. Interest then faded in artificial neural networks and they experienced a "winter" in the 1990s.
Amid the fluctuations in popular interest, Dr. Hinton continued to pursue research on neural networks with great diligence. In 1993, he introduced variational inference (a form of approximate Bayesian inference), for neural networks. In 2002, he introduced a fast learning algorithm for restricted Boltzmann machines (RBM) that allowed them to learn a single layer of distributed representation without requiring any labeled data. These methods allowed deep learning to work better and they led to the current deep learning revolution. In 2009, Dr. Hinton and two of his students used multilayer neural nets to make a major breakthrough in speech recognition that led directly to greatly improved speech recognition. In 2012, Dr. Hinton and two more students revolutionized computer vision by showing that deep learning worked far better than the existing state-of-the-art for recognizing objects in images.
To achieve their dramatic results, Dr. Hinton also invented a widely used new method called “dropout” which reduces overfitting in neural networks by preventing complex co-adaptations of feature detectors. He also invented “t-SNE” for visualizing high-dimensional data in a two-dimensional map. Of the countless AI-based technological services across the world, it is no exaggeration to say that few would have been possible without the results Dr. Hinton created.
AI has become widely used in various situations in our everyday lives, including image recognition by computers, audio responses by smart phones, experiments in self-driving vehicles and automated diagnosis of medical images. Since its birth 70 years ago, AI technology has finally gained a status that enables it to make major contributions to humankind. The achievements of Dr. Hinton make AI the means for creating a new society, and AI is expected to play an important role not only in creating safety and security in society, represented by the development of advanced transit systems, but also in resolving many global issues in the fields of energy and climate change that humankind must address. For these reasons, the Prize will be awarded to Dr. Geoffrey Hinton for his outstanding achievements.
- *1Deep learning: A machine learning method that employs neural networks, inspired by the neurons in the human brain, to allow a computer system to find structure in data automatically without human intervention.
- *2Eco-technology: a neologism combining imaging of the natural world (ecology), including civilization as a whole, and technology. Advocated by the Honda Foundation in 1979, it seeks new technological concepts required by human society in the sense of coexistence of people and technology.
- *3Backpropagation algorithm: An efficient procedure for computing how to change the connection strengths in a neural network so as to reduce the error in the network’s output.
Dr. Geoffrey E. Hinton
Professor Emeritus, the University of Toronto
Date of Birth, Place of Birth
December 1947, UK (British & Canadian citizenship)
Biography
1978–80: University of California, San Diego, Postdoctoral Fellow
1980–82: MRC Applied Psychology Unit, Cambridge, Research Scientist
1982–87: Carnegie Mellon University, Assistant & Associate Professor
1987–current: University of Toronto, Professor, Computer Science Department
1998–2001: University College London, Director, Gatsby Computational Neuroscience Unit
2013–current: Google Brain Team, Engineering Fellow, (half-time)
2016–current: Vector Institute, Chief Scientific Adviser (pro bono)
Major Awards Received
1998: Fellow of the Royal Society
2001: David E. Rumelhart Prize in Cognitive Science
2005: IJCAI Award for Research Excellence in Artificial Intelligence
2011: Herzberg Canada Gold Medal for Science and Engineering
2016: Foreign Member of American National Academy of Engineering
2016: IEEE/RSE James Clerk Maxwell Gold Medal
2016: BBVA Foundation of Knowledge Award, Information and Communication Technologies
2016: NEC C&C Award
2018: ACM Turing Award (with Yann LeCun and Yoshua Bengio)
Major Publications
A Learning Algorithm for Boltzmann Machines: (with Ackley, D. H. & Sejnowski, T. J.), Cognitive Science, Elsevier, 9 (1): 147–169, 1985
Learning Representations by Back-Propagating Errors: (with Rumelhart, D. E. & Williams, R.J.) Nature 323 (6088): 533–536, 9 October 1986
A Fast Learning Algorithm for Deep Belief Nets: (with Osindero, S. & Teh, Y.) Neural Computation, 18 (7): 1527-1554, July 2006
Reducing the Dimensionality of Data with Neural Networks: (with Salakhutdinov, R.R.) Science, 313 (5786): 504–507, 28 July 2006
ImageNet Classification with Deep Convolutional Neural Networks: (with Krizhevsky, A. & Sutskever, I.), NIPS 2012. Curran Associates Inc.: 1097–1105, 3 December 2012
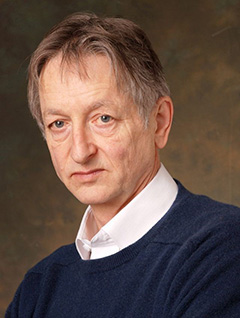